Environmental Impacts of Artificial Intelligence
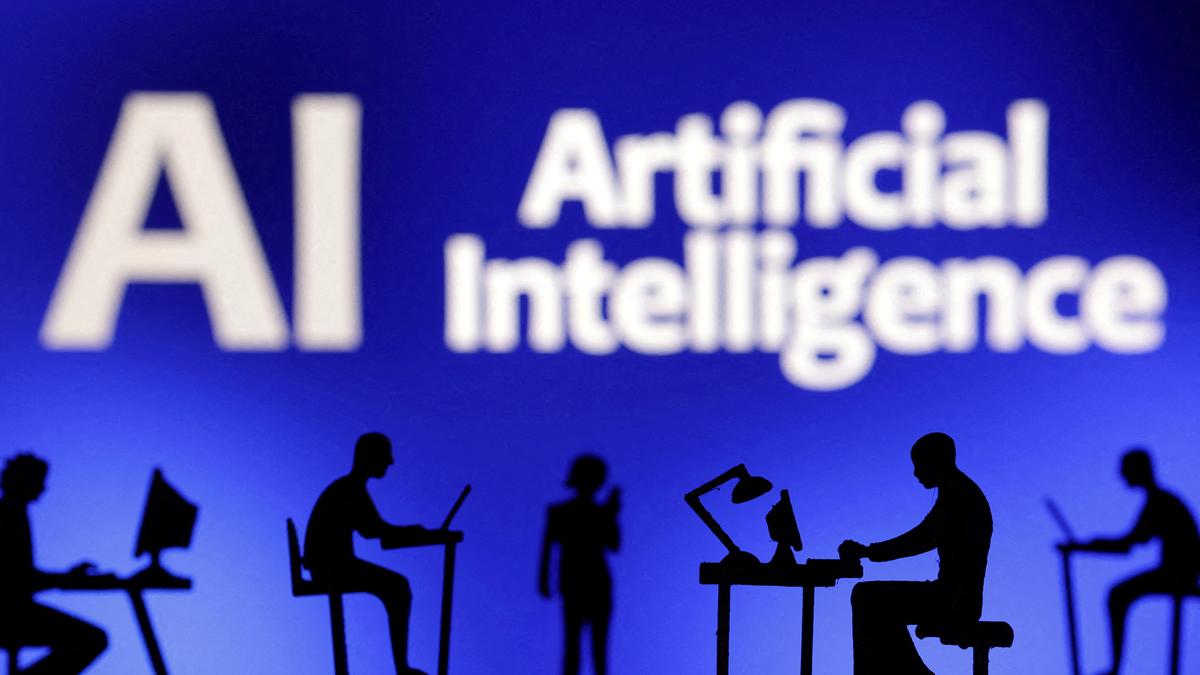
- 28 Feb 2025
Introduction
Artificial Intelligence (AI), encompassing technologies that simulate human thinking and decision-making, has become integral to contemporary life, influencing how we work, live, and conduct business. While AI’s foundational concepts date back to the 1950s, recent advances in computing power and data availability have fueled rapid growth. The global AI market, currently valued at $200 billion, is projected to contribute up to $15.7 trillion to the world economy by 2030. Major developments, such as the U.S.’s $500 billion Stargate Project and India's plans to build the world’s largest data centre in Jamnagar with Nvidia, highlight AI’s economic potential. However, this transformative technology also poses significant environmental challenges across its value chain.
Environmental Impact Across the AI Value Chain
The environmental footprint of AI emerges at multiple stages — from hardware production and model development to deployment and maintenance:
- Energy Consumption and Carbon Emissions:Data centres, the backbone of AI infrastructure, consume vast amounts of electricity, accounting for approximately 1% of global greenhouse gas emissions, a figure expected to double by 2026 (IEA). Advanced models like GPT-3 emit up to 552 tonnes of carbon dioxide equivalent during training, comparable to the annual emissions of dozens of cars. A single ChatGPT request consumes roughly ten times the energy of a standard Google search.
- E-Waste Generation and Resource Depletion:The rapid expansion of AI data centres exacerbates the e-waste crisis, with obsolete hardware often discarded irresponsibly. Manufacturing essential components like microchips demands rare earth minerals, mined through environmentally destructive processes.
- Water Usage:Data centres require millions of litres of water for cooling operations. Alarmingly, AI-related data centres are projected to consume six times more water than Denmark’s total usage, straining water resources, especially in regions already facing scarcity.
Global Awareness and Regulatory Efforts
Recognising the growing environmental burden, international organisations and governments have initiated efforts toward greener AI practices:
- At COP29, the International Telecommunication Union (ITU) underscored the urgent need for sustainable AI development.
- Regions like the European Union and the United States have introduced laws to mitigate AI’s environmental impact.
- However, despite over 190 countries adopting non-binding ethical AI guidelines, sustainability concerns remain largely absent from most national AI strategies.
Way Forward
To harmonise AI innovation with environmental responsibility, a multi-pronged approach is necessary:
- Clean Energy Adoption:Shifting to renewable energy sources for powering data centres and purchasing carbon credits are critical steps. Locating centres in regions rich in renewables can further lower carbon footprints.
- Efficiency in Hardware and Algorithms:Developing energy-efficient hardware and maintaining it regularly can substantially reduce emissions. Smaller, domain-specific models should be promoted to lessen computational demands.
- Optimisation of AI Systems:Studies by Google and the University of California, Berkeley, suggest that through optimised algorithms, specialised hardware, and efficient cloud data centres, the carbon footprint of large language models (LLMs) can be reduced by a factor of 100 to 1,000.
- Reusing Pre-trained Models:Businesses should prioritise fine-tuning existing models for new tasks over training fresh models from scratch, significantly saving energy.
- Measurement, Disclosure, and Accountability:Establishing global standards for tracking, measuring, and publicly disclosing the environmental impacts of AI systems is essential for fostering transparency and industry-wide accountability.
- Leveraging AI for Sustainability:AI itself can aid sustainability efforts, such as through projects like Google's DeepMind, which improves wind power forecasting, enabling better integration of renewable energy sources into national grids.
Conclusion
While AI holds immense promise for economic growth and societal advancement, it simultaneously presents critical environmental challenges. Integrating sustainability into the very design of the AI ecosystem is imperative. By embedding environmental responsibility at every stage—from model development to deployment—governments, businesses, and researchers can ensure that AI’s transformative potential is realised without compromising the planet’s future.